Alycia Holwerda
Hackathons

MUDAC19 Winning 2nd Place Data Visualization Award.

L-R: Alycia Holwerda, D.J. Hakimi, Eric Hanson, Christopher Zuelsdorf, Nayeem Imitiaz
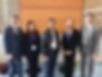
MUDAC Team '17

MUDAC19 Winning 2nd Place Data Visualization Award.
MUDAC 2017 Reflection
Data Derby 2017 Reflection
MUDAC 2018 Reflection
MUDAC 2019 Executive Summary
MUDAC 2019 Presentation
Data Derby 2019 Presentation
Throughout my undergraduate career I have competed in a total of five hackathons. A hackathon is a data science competition. A dataset is given to teams of 3-5 members to analyze and answer questions asked by the data sponsor. At the Midwest Undergraduate Data Analytic Competition (MUDAC) the data set was given at the event, and teams had under twenty-four hours to complete the competition. At the Advanced IT Data Derby, the data set was given a month in advance, and teams had a month to prepare presentations. I have competed at three MUDAC competitions and two Advanced IT competitions. These competitions follow the format of getting the data, figuring out what each variable means, cleaning the data, creating an accurate model, learning key insights, answering the data sponsors questions, creating a presentation, writing an executive summary, and presenting findings to judges. At the MUDAC competition I worked with data from Aeon, the Minnesota Wild, and the Water Quality Resource center. The Advanced IT competitions incorporated enormous datasets from the Rise Against Hunger nonprofit and then the Hired Heroes nonprofit. My teams have won awards for 1st place in the Advanced Category and Honorable mention at Advanced IT and 2nd place visualization award at MUDAC in 2019. Due to privacy concerns I can only speak of data and revelations about the Advanced IT hackathons and the MUDAC 2019 competition on the Water Resource Center data.
An important part of these competitions is to ethically use information (Information Literacy: Level 2). I cannot falsify findings since that would be incorrectly stating research. I cannot improperly use methods, because this would unethically be using information. Also, I cannot specifically talk about findings from private organizations such as Aeon and the Minnesota Wild whom do not share their data to the public. This would be unethically sharing their private data and assets.
While working at these competitions it was important to fully understand the questions being asked from the data sponsors. These were the key points that the data sponsors wanted to learn from having their data analyzed. For example, the Hired Heroes data sponsor wanted to know what type of veterans were using their services, which services were most effective, who was donating more to the nonprofit among other questions. My team had to identify research questions in each hackathon to address and how we were going to answer them (Original Research: Level 1).
The next step was to actually figure out how we would answer these questions. We would identify variables given in the data that might help solve questions. For example, in the Water quality data we looked at each individual variable and decided which were important such as suspended solids amount, location, slope of field, and soil type. We were able to develop the research question more by figuring out what specific data we should target to answer the questions in a short time frame (Original Research: Level 2).
After completely understanding the business questions and the data variables, my team would begin by cleaning the data. This means handing missing values, getting rid of outliers, seeing which variables were useable, and changing data types of variables. After that we would always have to create a predictive model. In all three MUDAC hackathons, a model was wanted. For example, we needed to predict what variables led to high total suspended solids content in soil. I tried various modeling techniques such as forward, backward, and stepwise selection for regression models. I tried binary trees, random forests, neural networks, and logistic models for binary classifications. We used various technologies when working with the data such as Python, R, SAS, excel, and Tableau. We created many different visualizations with Tableau to further understand the data. From these various methods we were able to conclude many key facts. For example, a key take away we had from the Water Quality data was that areas with high farmland content had high total suspended solids and thus worse water quality. An important trait of successful data analysist is being able to translate statistics into valuable insights and advice for businesses. For example, when we learned farmland had worse water quality, we suggested that the water resource center give notice to farmers to use better pesticides for the environment. However, not everything went perfect in these competitions. We did struggle for hours on how to improve a faulty model and determine, why the model was performing so poorly. Sometimes we decided to include these struggles into our presentation. For example, we thought slope of soil would have a bigger impact on water quality since a greater slope equals more runoff. We noticed that the slope variable was covering too wide of a range and thus the average percent of slope was over a large area. So, a different method for analyzing slope should have been given. Through these hackathons I was able to learn so much not only about the data but also the research process. These hackathons allowed me to conduct primary research that related to statistics (Original Research: Level 3).
When competing in a hackathon, evaluating and incorporating selected information is a key ability (Information Literacy: Level 3). A data analyst could have spent years working with the data from Rise Against Hunger since it was multiple data sets with millions of rows and hundreds of columns. My team learned so much from this data that we could have presented for hours. However, the data sponsor would not think everything we discovered was useful information. We had to select and incorporate the key information that the data sponsor could actually use. When completing a hackathon, we were able to use information effectively and ethically to accomplish a specific research goal (Information Literacy: Level 4).
The final step in a hackathon was presenting results. All the competitions wanted a two-page executive summary as well as a 5-10 minute presentation (Dissemination of Results: Level 2). As a team we had to decide which information was the most valuable and what was the best method of presenting these findings to the judges. At these hackathons we exhibited or completed research (Original Research: Level 4).
When working at these hackathons we presented our results. We could present our results at different venues such as online on GitHub or the dataset website. The best venue would be to the actual data sponsors (Dissemination of Results: Level 1). At MUDAC competitions, we actually had the opportunity to present our key findings to the data sponsors. For example, when working with the water quality data we presented our findings to actual water quality workers (Dissemination of Results: Level 3). MUDAC was a peer- reviewed venue where we were able to submit the results (Dissemination of Results: Level 4). Experts in the field were able to hear our key findings, speak with us on how to improve our research, and address what they felt was very important. The main takeaway I have from presenting our findings is to decide the key points and communicate them in terms of the business sponsors so they can actually improve the organization. Also, when hearing other teams’ presentations, I found it very valuable in understanding more about the research process and different methods to analyze the data. For example, certain teams took a more business approach to the Water Quality Data while others used different statistical models such as Bayes. Through hackathons I was able to reflect upon how my project led to new knowledge and understanding for the research process (Information Synthesis: Level 4).
I loved competing in hackathons. I loved being able to address business needs, research more information, and identify key solutions to answers proposed by data sponsors. It’s a good thing that this will be my career. I cannot wait to analyze more data for IBM when I work there starting this January. In my spare time I hope to continue to do hackathons online, and maybe win some price money. In conclusion, I hope to continue to learn and to help the world more through data.
Image powered by D.R.E.A.M. Twitter account @MNSU_DREAM